Summary: Researchers developed a human-inspired AI training method that improves object recognition by incorporating spatial data, leading to a 14.99% performance increase. This new approach mimics the way infants learn from their environments, thereby training AI systems more effectively. The results could advance AI for extreme environments or space exploration.
Highlights:
- New AI training method mimics infant learning with spatial data.
- AI models trained with this method outperformed baseline models by up to 14.99%.
- The method was tested in virtual environments to simulate real-world learning.
Source: State of Pennsylvania
A new human-inspired approach to training artificial intelligence (AI) systems to identify objects and navigate their surroundings could pave the way for developing more advanced AI systems to explore extreme environments or worlds far away, according to research by an interdisciplinary team at Penn State.
During the first two years of life, children experience a somewhat restricted set of objects and faces, but from many different viewpoints and under varying lighting conditions.
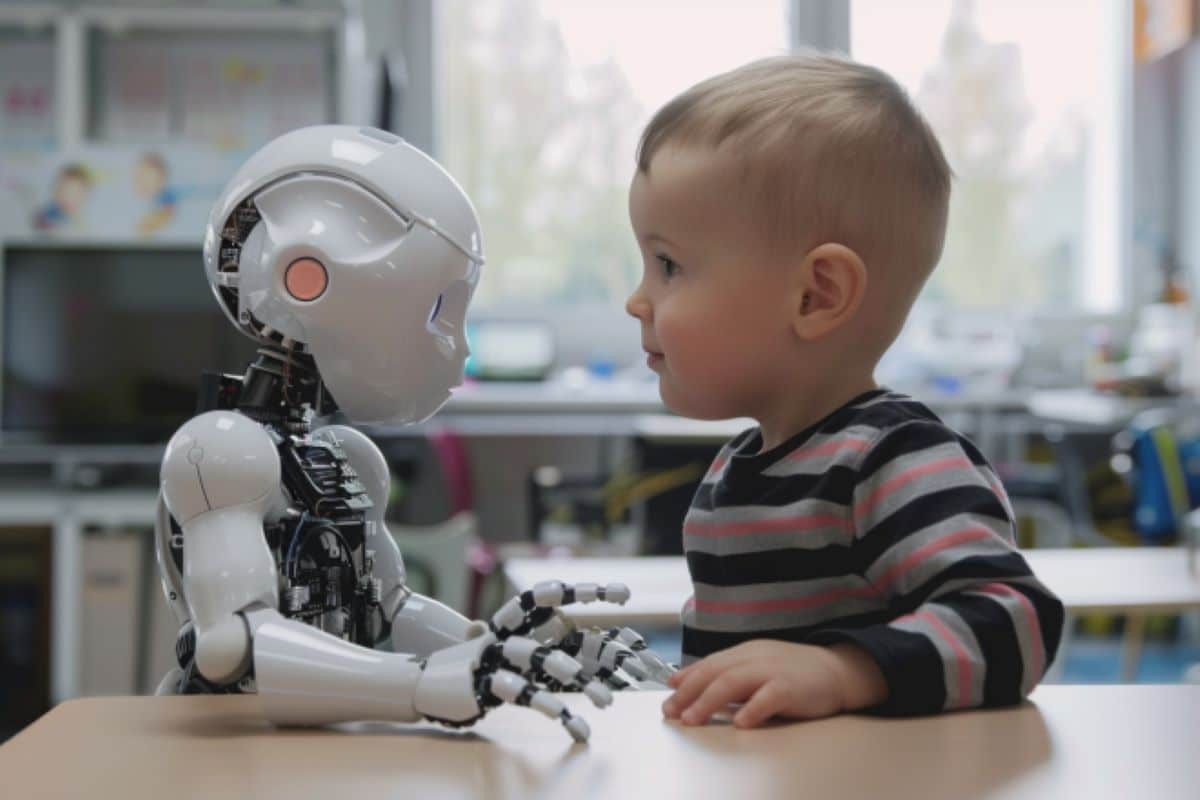
Inspired by this developmental vision, researchers introduced a new machine learning approach that uses spatial position information to train AI visual systems more effectively. They found that AI models trained using the new method outperformed baseline models by up to 14.99%.
They reported their findings in the May issue of the journal Patterns.
“Current AI approaches use massive sets of random photographs from the Internet for training purposes. Instead, our strategy draws on developmental psychology, which studies how children perceive the world,” said Lizhen Zhu, lead author and doctoral student in Penn State’s College of Information Science and Technology.
The researchers developed a new contrastive learning algorithm, which is a type of self-supervised learning method in which an AI system learns to detect visual patterns to identify when two images are derivations of the same image from base, which gives rise to a positive pair. However, these algorithms often treat images of the same object taken from different angles as separate entities rather than positive pairs.
Taking into account environmental data, including location, allows the AI system to overcome these challenges and detect positive pairs regardless of changes in camera position or rotation, angle or camera condition. lighting and focal length, or zoom, according to the researchers.
“We hypothesize that infants’ visual learning depends on location perception. In order to generate an egocentric dataset with spatio-temporal information, we implemented virtual environments in the ThreeDWorld platform, which is an interactive, high-fidelity 3D physics simulation environment. This allowed us to manipulate and measure the location of the viewing cameras as if a child were walking through a house,” Zhu added.
The scientists created three simulation environments: House14K, House100K, and Apartment14K, with “14K” and “100K” referring to the approximate number of image samples taken in each environment. Then they ran basic contrastive learning models and models with the new algorithm through the simulations three times to see how well each classified the images.
The team found that models trained on their algorithm outperformed baseline models on a variety of tasks. For example, during a room recognition task in the virtual apartment, the augmented model performed on average 99.35%, an improvement of 14.99% compared to the base model.
These new datasets are available for other scientists to use in their training through www.child-view.com.
“It is always difficult for models to learn in a new environment with a small amount of data. Our work represents one of the first attempts at more energy-efficient and flexible AI training using visual content,” said James Wang, distinguished professor of information science and technology and Zhu’s advisor.
The research has implications for the future development of advanced AI systems intended to navigate and learn new environments, according to the scientists.
“This approach would be particularly beneficial in situations where a team of autonomous robots with limited resources must learn to navigate a completely unfamiliar environment,” Wang said.
“To pave the way for future applications, we plan to refine our model to better exploit spatial information and incorporate more diverse environments.”
Collaborators from Penn State’s Department of Psychology and Department of Computer Science and Engineering also contributed to this study.
Funding: This work was supported by the US National Science Foundation, as well as the Institute for Computational and Data Sciences at Penn State.
About this AI research news
Author: Francisco Tutella
Source: State of Pennsylvania
Contact: Francisco Tutella – Penn State
Picture: Image is credited to Neuroscience News
Original research: Free access.
“Integrating Simulated Spatial Context Information Improves the Effectiveness of Contrastive Learning Models” by Lizhen Zhu et al. Patterns
Abstract
Integrating simulated spatial context information improves the effectiveness of contrastive learning models
Strong points
- Creation of an approach that uses spatial context as a similarity signal
- Method of constructing image datasets using an environmental sampling agent
- Training with contextual information improves the state of the art in contrastive learning
- Simulated data provides new forms of physically realistic augmentations
The big picture
Despite being trained on large datasets, current computer vision systems lag behind human children in learning the visual world.
One possible reason for this discrepancy is that humans actively explore their environments as embodied agents, sampling data from a stable visual world accompanied by context.
Somewhat resembling the human childhood experience, contrastive learning is a machine learning technique that allows the learning of general features without having labeled data.
This is done by grouping similar things or objects together and separating those that are different. Contrastive learning methods can be applied to multiple tasks, for example to train visual learning agents.
Improving these machine learning strategies is important for the development of effective intelligent agents, such as robots or vehicles, that can explore and learn from their environment.