Summary: can predict whether a person with mild cognitive impairment will develop Alzheimer’s disease with 78.5% accuracy by analyzing speech. This tool could enable earlier diagnoses and more accessible screening for cognitive impairment without expensive tests.
The model uses machine learning to assess speech content, providing a non-invasive way to monitor dementia risk. Further research aims to improve and expand this technology.
Highlights:
- High accuracy: AI model predicts Alzheimer’s disease progression with 78.5% accuracy.
- Accessible screening: Could make dementia diagnosis easier and more widely available.
- Speech analysis: analyzes speech content, not just acoustic characteristics.
Source: Boston University
Trying to determine whether someone has Alzheimer’s disease usually involves a battery of evaluations: interviews, brain imaging, blood and spinal fluid tests. But by then, it’s probably already too late: memories have begun to fade, long-established personality traits have begun to subtly change.
If detected early, innovative new treatments can slow the disease’s relentless progression, but there is no foolproof way to predict who will develop Alzheimer’s-associated dementia.
Now, researchers at Boston University say they have designed a promising new computer program, or artificial intelligence model, that could one day help change that situation, simply by analyzing a patient’s speech.
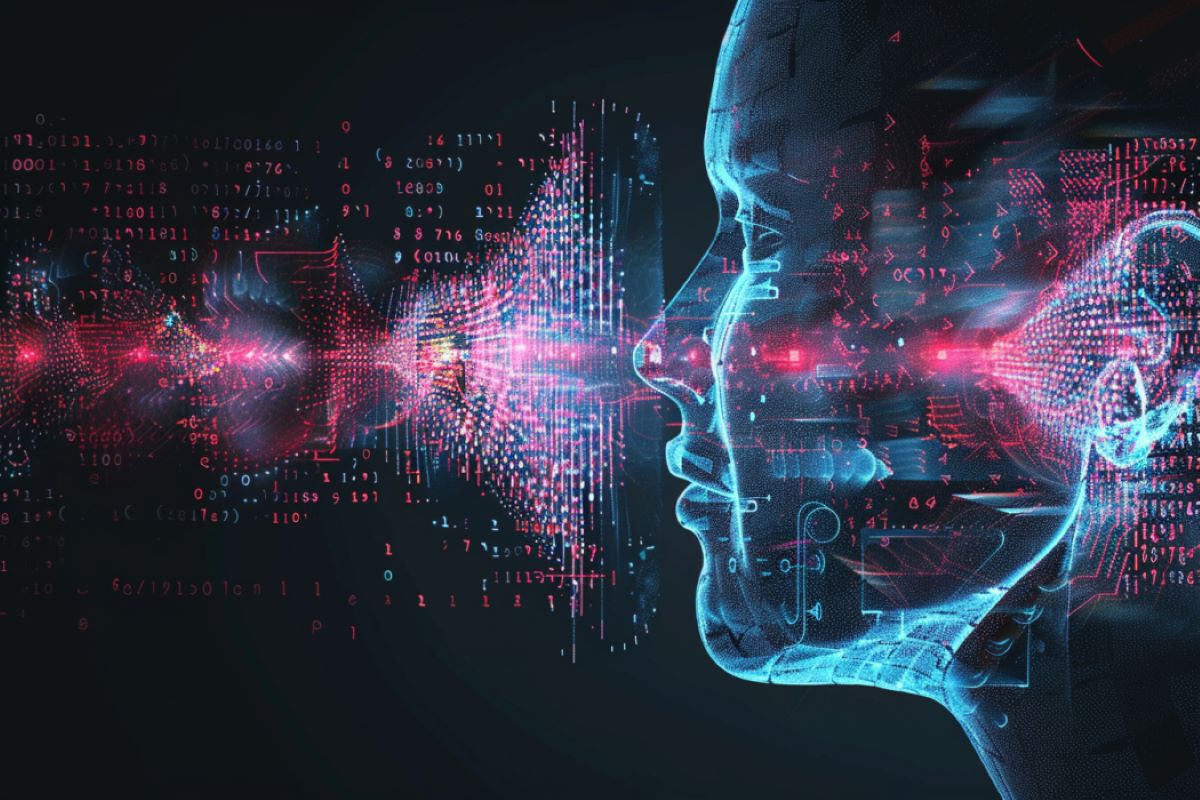
Their model can predict, with an accuracy rate of 78.5 percent, whether a person with mild cognitive impairment is likely to remain stable over the next six years or fall into dementia associated with Alzheimer’s disease.
While allowing clinicians to look ahead and make earlier diagnoses, the researchers say their work could also help make cognitive impairment screening more accessible by automating parts of the process: no costly lab tests , imaging test or even office visit is required.
The model is powered by machine learning, a subset of AI in which computer scientists teach a program to analyze data independently.
“We wanted to predict what would happen over the next six years and we found that we could reasonably make this prediction with relatively good confidence and accuracy,” says Ioannis (Yannis) Paschalidis, director of the Rafik B Institute. Hariri of Computer Science and Informatics of the BU. Computer science and engineering.
“This shows the power of AI. »
The multidisciplinary team of engineers, neurobiologists and computer and data scientists published their results in Alzheimer’s and dementiathe journal of the Alzheimer’s Association.
“We hope, like everyone else, that there will be more and more treatments for Alzheimer’s disease available,” says Paschalidis, professor emeritus of engineering in the BU College of Engineering and founding member of the Faculty of computer science and data sciences.
“If you can predict what’s going to happen, you have a greater opportunity and a window of time to intervene with medications, and at least try to keep the disease stable and prevent the transition to more severe forms of dementia.”
Calculate the probability of developing Alzheimer’s disease
To train and build their new model, the researchers turned to data from one of the nation’s oldest and longest-running studies, the BU-led Framingham Heart Study.
Although the Framingham Study focuses on cardiovascular health, participants with signs of cognitive decline undergo regular neuropsychological testing and interviews, producing a wealth of longitudinal information about their cognitive well-being.
Paschalidis and his colleagues received audio recordings of 166 initial interviews with people, ages 63 to 97, diagnosed with mild cognitive impairment — 76 who would remain stable over the next six years and 90 whose cognitive function would gradually decline.
They then used a combination of speech recognition tools (similar to the programs that power your smart speaker) and machine learning to train a model that could detect links between speech, demographics, diagnosis and progression of the disease. After training it on a subset of the study population, they tested its predictive prowess on the rest of the participants.
“We combine the information we extract from the audio recordings with very basic demographic data (age, gender, etc.) and we get the final score,” says Paschalidis. “You can think of the score as the likelihood that a person will remain stable or progress to dementia. It had significant predictive ability.
Rather than using the acoustic characteristics of speech, such as enunciation or speed, the model simply relies on the content of the interview: the words spoken, the way they are structured.
And Paschalidis says the information they put into the machine learning program is rough: The recordings, for example, are messy – low quality and full of background noise.
“It’s a very relaxed recording,” he says. “And yet, with this dirty data, the model is able to make something out of it.”
This is important because the project aimed in part to test AI’s ability to make the dementia diagnosis process more efficient and automated, with little human involvement.
In the future, the researchers say, models like theirs could be used to provide care to patients who are not near medical centers or to provide routine monitoring through interaction with an at-home app, increasing thus considerably increasing the number of people screened.
According to Alzheimer’s Disease International, the majority of people with dementia worldwide never receive a formal diagnosis, leaving them without treatment and care.
Rhoda Au, co-author of the paper, says AI has the power to create “equality of opportunity in science and healthcare.” The study builds on previous work by the same team, in which they found that AI could accurately detect cognitive impairments using voice recordings.
“Technology can overcome the stigma of work that can only be done by those with resources or care that relies on specialized expertise that is not accessible to everyone,” says Au, professor of anatomy and in neurobiology at the BU Chobanian & Avedisian School of Medicine. .
For her, one of the most exciting discoveries was “that a cognitive assessment method with the potential to be as inclusive as possible – possibly independent of age, sex/gender, education, language, culture, income, geography – could serve as a potential method. screening tool to detect and monitor symptoms related to Alzheimer’s disease.
A diagnosis of dementia at home
In his future research, Paschalidis would like to explore using data from not only formal clinician-patient interviews — with their written questions and predictable back-and-forths — but also from more natural, everyday conversations.
He’s already planning a project to determine whether AI can help diagnose dementia through a smartphone app, as well as expanding the current study beyond speech analysis (Framingham’s tests also include patient drawings and daily life pattern data) to improve the predictive accuracy of the model. .
“Digital is the new blood,” says Au. “You can collect it, analyze it for what is known today, store it, and reanalyze it for whatever emerges tomorrow.”
Funding: This research was supported, in part, by the National Science Foundation, the National Institutes of Health, and the BU Rajen Kilachand Fund for Integrated Life Science and Engineering.
About this research news on AI and Alzheimer’s disease
Author: Catherine Gianni
Source: Boston University
Contact: Katherine Gianni – Boston University
Picture: Image is credited to Neuroscience News
Original research: Free access.
“Prediction of the progression of Alzheimer’s disease within 6 years using speech: a new approach exploiting language models” by Ioannis Paschalidis et al. Alzheimer’s and dementia
Abstract
Predicting the progression of Alzheimer’s disease over 6 years using speech: a new approach exploiting language models
INTRODUCTION
Identifying individuals with mild cognitive impairment (MCI) who are at risk of developing Alzheimer’s disease (AD) is crucial for early intervention and clinical trial selection.
METHODS
We applied natural language processing techniques along with machine learning methods to develop a method for automated prediction of progression to AD within 6 years using speech. The study design was evaluated based on neuropsychological testing interviews of not = 166 participants from the Framingham Heart Study, including 90 cases of progressive MCI and 76 cases of stable MCI.
RESULTS
Our best models, which used features generated from voice data, as well as age, gender and education level, achieved an accuracy of 78.5% and a sensitivity of 81.1% in predicting the progression from MCI to AD within 6 years.
DISCUSSION
The proposed method offers a fully automated procedure, offering the possibility of developing an inexpensive, widely accessible and easy to administer screening tool for the prediction of progression from MCI to AD, thus facilitating the development of remote assessment.