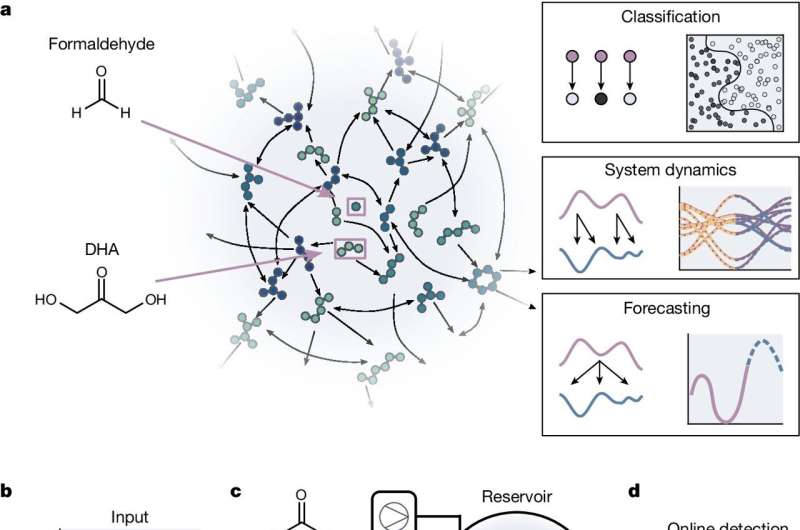
Schematic overview of the Formosa reservoir computer. Credit: Nature (2024). DOI: 10.1038/s41586-024-07567-x
Researchers at the Institute of Molecules and Materials at Radboud University in the Netherlands have demonstrated that a complex network of self-organizing chemical reactions can perform various computational tasks, such as nonlinear classification and prediction of complex dynamics.
The field of molecular computing is of interest to researchers who want to exploit the computing power of chemical and biological systems. In these systems, chemical reactions or molecular processes act as a reservoir computer, transforming inputs into high-dimensional outputs.
The research, published in Naturewas led by Professor Wilhelm Huck of Radboud University.
Researchers have exploited the potential of chemical and biological networks because of their complex computational capabilities. However, implementing molecular computing presents engineering and design challenges.
Rather than trying to design molecular systems to perform specific computing tasks, Professor Huck and his team are studying how naturally complex chemical systems can exhibit emergent computing properties.
“I am very interested in the chemical forces that led to the origin of life. In this context, we are looking for mechanisms by which chemical evolution can shape the properties of complex reaction mixtures. This research has led us to think about how molecular systems can process information,” he told Phys.org.
The formose reaction
The formose reaction is a chemical reaction that synthesizes sugars from formaldehyde in the presence of a catalyst, calcium hydroxide. This reaction was chosen because of its unique properties.
Professor Huck explains: “Although chemistry may seem complex to the uninitiated, most reaction sequences are quite linear. The formose reaction is the only example of a self-organizing reaction network with a highly nonlinear topology, containing many positive and negative feedback loops.”
In other words, the reaction is not simple and produces several intermediate compounds that in turn react to form new compounds. These dynamic reactions can give rise to a diverse set of chemical species and are non-linear in nature.
Additionally, the network includes positive feedback loops that amplify the outcomes of reactions and negative feedback loops that attenuate the outcomes of reactions.
The network is said to be “self-organizing” because it evolves naturally and responds to chemical inputs without requiring external intervention, producing a diverse range of results.
Computational capabilities emerge from inherent properties of the network rather than being explicitly programmed, making computation very flexible.
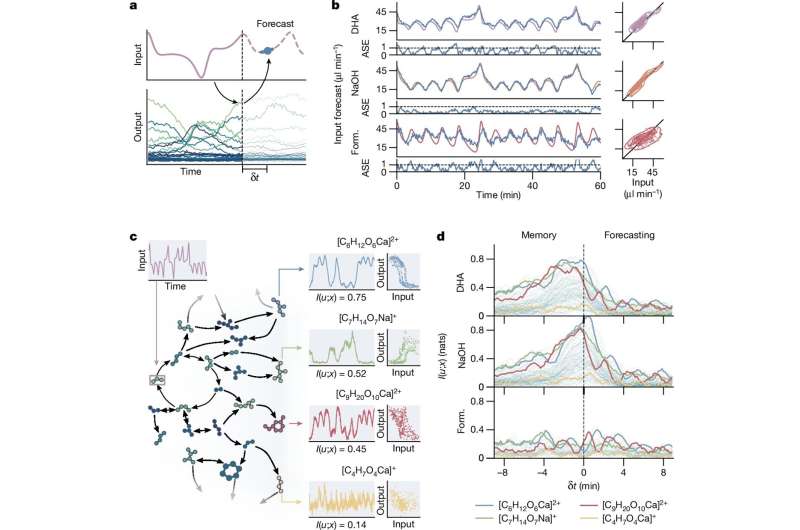
Memory and prediction in the formosa reservoir computer. Credit: Nature (2024). DOI: 10.1038/s41586-024-07567-x
Implementation of the tank computer
The researchers used a continuous stirred tank reactor (CSTR) to implement the formose reaction. The input concentrations of four reactants (formaldehyde, dihydroxyacetone, sodium hydroxide, and calcium chloride) are controlled to modulate the behavior of the reaction network.
The output molecule is identified using a mass spectrometer, which can track up to 106 molecules. This setup can be used to perform calculations, with the reactant concentrations being the input value to any function to be calculated.
But first, the system must be trained to find the result of this calculation, which is done using a set of weights.
“We need to find a set of weights that converts the traces in the mass spectrometer into the correct value for the calculation. This is a linear regression problem and is simple to calculate. Once this is done, the tank computer calculates the result of this function for any new input,” explained Professor Huck.
Weights are coefficients that determine how much influence each input has on the output. This training step is essential because it allows the reservoir to learn and predict how input changes affect the output so that it can predict the output for a new set of inputs.
Computing capabilities
The researchers used the reservoir computer to perform several tasks. The first was to perform nonlinear classification tasks. The reservoir computer could emulate all Boolean logic gates and even tackle more complex classifications like XOR, checkers, circles, and sine functions.
The team also showed that they could predict the behavior of a complex metabolic network model of E. coli, accurately capturing both linear and nonlinear responses to fluctuating inputs over different concentration ranges.
Additionally, the system demonstrated its ability to predict future states of a chaotic system (the Lorenz attractor), accurately predicting two of the three input dimensions several hours in advance.
The research team also discovered that some chemical species in the system exhibit short-term memory, retaining information about past inputs.
They also demonstrated a proof of concept for an all-chemical readout using colorimetric reactions, showing how the state of the system could be interpreted without electronic measuring devices.
In other words, the state of the system could be interpreted using the color changes resulting from chemical reactions, eliminating the need for electronic measuring devices.
Origins of life, neuromorphic computing and beyond
This new approach to molecular computing could bridge the gap between artificial systems and the information processing capabilities of living cells.
It suggests a more scalable and flexible approach to molecular computing, opening up possibilities for creating autonomous chemical systems capable of processing information and responding to their environment without external electronic control.
Professor Huck expressed his team’s interest in this area, saying: “Can we integrate reservoir computing into chemical systems that sense their environment, process that information and take appropriate action?
“This would require coupling the reservoir to other elements capable of translating the brain’s chemical production into a form of mechanical response or an interaction with living cells, for example.”
This research also has interesting implications for the origin of life. The emerging computational properties of this relatively simple chemical system could provide insights into how early biological systems may have evolved information-processing capabilities.
Professor Huck mentioned that this was his main motivation for studying reservoir calculation.
The research team also sees potential in neuromorphic computing, which mimics the neural structure and functioning of the human brain to improve efficiency and computing power.
“We are very interested in exploring the technological limits of the computing power of the Formosa Reservoir Computer – this is ongoing research in collaboration with IBM Zurich. Reservoir computing is an example of neuromorphic computing, which is of interest because it is expected to consume less energy than conventional computers,” says Professor Huck.
More information:
Mathieu G. Baltussen et al, Chemical reservoir calculation in a self-organized reaction network, Nature (2024). DOI: 10.1038/s41586-024-07567-x
© 2024 Science X Network
Quote:Scientists demonstrate chemical reservoir calculation using formose reaction (2024, July 13) retrieved July 14, 2024 from https://phys.org/news/2024-07-scientists-chemical-reservoir-formose-reaction.html
This document is subject to copyright. Apart from any fair dealing for the purpose of private study or research, no part may be reproduced without written permission. The content is provided for informational purposes only.