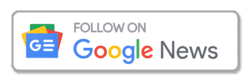
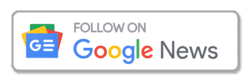
Researchers at Boston University have created a revolutionary artificial intelligence (AI) model that can predict whether a person with mild cognitive impairment will develop Alzheimer’s disease within six years, based solely on their speech. The model showed an impressive accuracy rate of 78.5%, providing a non-invasive and accessible method for early diagnosis. The results were published in the journal Alzheimer’s and dementia.
Alzheimer’s disease is the most common cause of dementia and typically progresses over a long period of time with only mild cognitive changes apparent. People with mild cognitive impairment are at higher risk of developing Alzheimer’s disease, with annual conversion rates ranging from 3% to 15%.
Being able to quickly and accurately predict who will progress from mild cognitive impairment to Alzheimer’s disease is critical to early treatment and inclusion in clinical trials of new drugs. Traditional methods of diagnosing Alzheimer’s disease, such as brain imaging and cerebrospinal fluid testing, are invasive, expensive, and not readily available in all regions. In contrast, speech analysis during neuropsychological testing is a less invasive and potentially more scalable approach.
The Boston University researchers conducted their study using data from the Framingham Heart Study, which has been recording neuropsychological testing interviews since 2005. The study cohort consisted of 166 people with cognitive impairment, including 59 men and 107 women, with a median age of 81. These participants underwent neuropsychological testing designed to assess various cognitive domains such as memory, language, visuospatial skills, abstract reasoning, and attention. Each testing session, which lasted about an hour, was audio recorded and stored in .wav format.
The researchers transcribed the audio recordings into text using automated speech recognition software. Each utterance was then logged, i.e. assigned to the participant or examiner, and categorized into specific subtests such as the Boston Naming Test or the Wechsler Memory Scale. The text data was processed using the Universal Sentence Encoder, a deep learning model that transforms text into numerical vectors representing semantic content.
To predict whether individuals with mild cognitive impairment would progress to Alzheimer’s disease within six years, the researchers used logistic regression models. They generated embedding vectors from the transcribed text and trained the models on these vectors, as well as demographic information such as age, gender, and education level. Model performance was assessed using stratified group k-fold cross-validation, ensuring that the data were split into multiple folds for training and testing to fully validate the results.
The AI model developed by the researchers achieved an accuracy rate of 78.5% and a sensitivity of 81.1% in predicting progression from mild cognitive impairment to Alzheimer’s disease within six years. Sensitivity refers to the model’s ability to correctly identify people who will progress to Alzheimer’s disease, while specificity, at 75%, measures its accuracy in identifying those who will not progress. These results indicate strong predictive power, particularly in identifying future Alzheimer’s patients.
The analysis found that speech features extracted from neuropsychological test recordings were strong predictors of disease progression. The inclusion of text features alone outperformed traditional neuropsychological test results and demographic factors. This suggests that subtle changes in speech patterns and language use can provide valuable information about cognitive decline, even before more obvious symptoms appear.
“We wanted to predict what would happen in the next six years, and we found that we could reasonably make that prediction with relatively good confidence and accuracy,” said Ioannis (Yannis) Paschalidis, director of the Rafik B. Hariri Institute for Computer Science and Computational Science and Engineering at Boston University. “That shows the power of AI.”
Interestingly, the study also found that older women, people with lower levels of education, and those carrying specific genetic markers, such as the apolipoprotein E gene allele, were more likely to develop Alzheimer’s disease. These findings are consistent with existing research on risk factors for Alzheimer’s disease, reinforcing the validity of the AI model’s predictions.
Although the AI model is promising, it has limitations that should be considered. The study cohort was predominantly white, limiting the generalizability of the results to more diverse populations. The specificity of the model, while reasonable, still leaves room for improvement to reduce the cost of clinical trials by better identifying candidates for new treatments.
Additionally, the model relies on speech data, meaning that variations in dialect, language proficiency, and cultural differences could impact its accuracy. Future research should aim to validate these findings on more diverse and larger populations and explore the inclusion of other types of data, such as patient drawings and daily living habits, to improve predictive accuracy.
As study co-author Rhoda Au points out, AI has the potential to create “a level playing field in science and healthcare,” overcoming bias and resource limitations. The technology can democratize access to early diagnosis and treatment, making it accessible to a broader population.
“Technology can help overcome the stigma of work that can only be done by those with the resources, or of care that relies on specialized expertise that is not available to everyone,” said Au, a professor of anatomy and neurobiology. One of the most exciting findings was “that a cognitive assessment method that has the potential to be as inclusive as possible—perhaps independent of age, gender, education, language, culture, income, geography—could serve as a potential screening tool to detect and monitor symptoms related to Alzheimer’s disease.”
The researchers plan to expand their study to include data from more natural conversations, rather than just structured neuropsychological tests, possibly via a smartphone app. This approach could make the AI model even more accessible and practical for large-scale use. In addition, they want to incorporate other types of data to further improve the model’s accuracy.
“Digital is the new blood,” Au explains. “We can collect it, analyze it to know what we know today, store it and reanalyze it to know what will emerge tomorrow.”
“We hope, like everyone else, that more and more treatments for Alzheimer’s disease will become available,” Paschalidis added. “If we can predict what’s going to happen, we have more opportunities and time to intervene with medications and at least try to maintain the stability of the disease and prevent the transition to more severe forms of dementia.”
The study, “Predicting Alzheimer’s Disease Progression Within 6 Years Using Speech: A Novel Approach Exploiting Language Models,” was authored by Samad Amini, Boran Hao, Jingmei Yang, Cody Karjadi, Vijaya B. Kolachalama, Rhoda Au, and Ioannis C. Paschalidis.