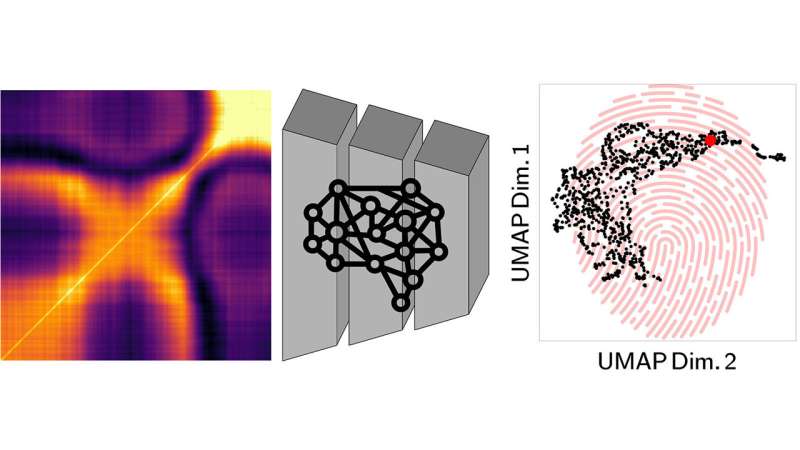
The AI-NERD model learns to produce a unique fingerprint for each XPCS data sample. Mapping fingerprints from a large experimental data set can identify trends and repeating patterns that help us understand how materials evolve. Credit: Argonne National Laboratory
Just like humans, materials change over time. They also behave differently when stressed or relaxed. Scientists looking to measure the dynamics of material change have developed a new technique that leverages X-ray photon correlation spectroscopy (XPCS), artificial intelligence (AI), and machine learning.
This technique creates “fingerprints” of different materials that can be read and analyzed by a neural network to gain new insights that scientists previously had no access to. A neural network is a computer model that makes decisions in a similar way to the human brain.
In a new study by researchers at the Advanced Photon Source (APS) and the Center for Nanoscale Materials (CNM) at the U.S. Department of Energy’s (DOE) Argonne National Laboratory, scientists paired XPCS with an unsupervised machine learning algorithm, a form of neural network that requires no specialized training. The algorithm teaches itself to recognize hidden patterns in arrangements of X-rays scattered by a colloid, a group of particles suspended in a solution. APS and CNM are DOE Office of Science user facilities.
An article based on the study appears in Nature Communications.
“The way we understand how materials move and change over time is to collect X-ray scattering data,” said Argonne postdoctoral researcher James (Jay) Horwath, first author of the study.
These patterns are too complex for scientists to detect without the help of AI.
“When we project the X-ray beam, the patterns are so diverse and complex that it becomes difficult, even for experts, to understand what they mean,” Horwath said.
For researchers to better understand what they are studying, they need to condense all the data into fingerprints that contain only the most essential information about the sample.
“You can think of it as having the genome of the material; it contains all the information needed to reconstruct the entire picture,” Horwath said.
The project is called Artificial Intelligence for Non-Equilibrium Relaxation Dynamics, or AI-NERD. The fingerprints are created using a technique called an autoencoder. An autoencoder is a type of neural network that transforms the original image data into a fingerprint (called a latent representation by scientists) and also includes a decoding algorithm used to get from the latent representation to the full image.
The researchers’ goal was to try to create a fingerprint map of the material, grouping fingerprints with similar characteristics into neighborhoods. By looking holistically at the characteristics of different fingerprint neighborhoods on the map, the researchers were able to better understand how the materials were structured and how they changed over time under stress and relaxation.
In simple terms, AI has good general pattern recognition capabilities, which allows it to efficiently categorize different X-ray images and sort them on the map.
“The goal of AI is simply to treat scatter patterns like regular images or photos and digest them, to determine what the repeating patterns are,” Horwath said. “AI is an expert in pattern recognition.”
Using AI to understand broadcast data will be particularly important as the APS comes online. The upgraded facility will generate X-ray beams 500 times brighter than the original APS.
“The data we get from the upgraded APS will need the power of AI to sort through it,” Horwath said.
The CNM theory group collaborated with the computational group in Argonne’s X-ray Sciences Division to perform molecular simulations of the polymer dynamics demonstrated by XPCS and, in the future, synthetically generate data for training AI workflows like AI-NERD.
The study’s authors are James (Jay) Horwath, Xiao-Min Lin, Hongrui He, Qingteng Zhang, Eric Dufresne, Miaoqi Chu, Subramanian Sankaranaryanan, Wei Chen, Suresh Narayanan and Mathew Cherukara of Argonne. Chen and He have joint appointments at the University of Chicago, and Sankaranaryanan has a joint appointment at the University of Illinois at Chicago.
More information:
James P. Horwath et al, AI-NERD: Elucidating Beyond-Equilibrium Relaxation Dynamics Using AI-Informed X-Ray Photon Correlation Spectroscopy, Nature Communications (2024). DOI: 10.1038/s41467-024-49381-z
Provided by Argonne National Laboratory
Quote:Scientists develop new AI method to create material ‘fingerprints’ (2024, July 16) retrieved July 17, 2024 from https://phys.org/news/2024-07-scientists-ai-method-material-fingerprints.html
This document is subject to copyright. Apart from any fair dealing for the purpose of private study or research, no part may be reproduced without written permission. The content is provided for informational purposes only.