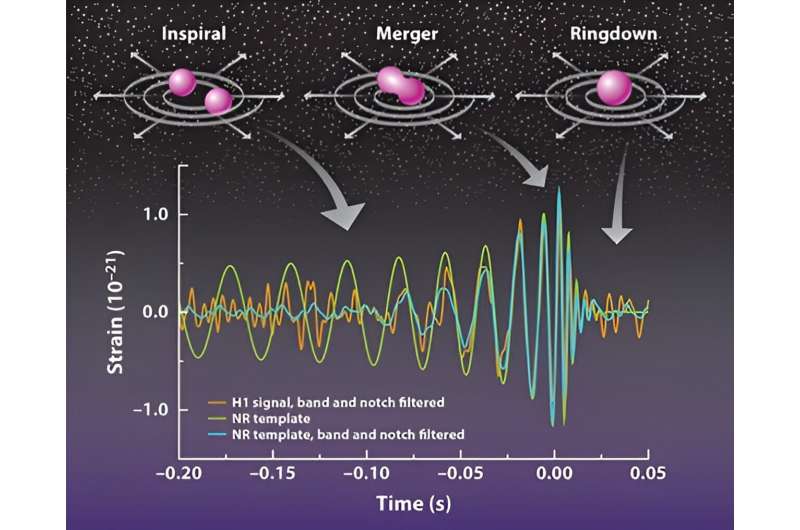
The gravitational wave signal received by a LIGO detector (orange), overlaid by theoretical predictions of general relativity (green) and the appearance of the expected signal in the detector (blue). Credit: Physics magazine, APS (https://physics.aps.org/articles/v9/52)
Since the direct detection of gravitational waves in 2015, scientists have had to resort to some tinkering: they can only detect waves that match theoretical predictions, which is quite the opposite of how science is usually conducted.
A group of physicists has developed a computer model that can capture all the gravitational waves that pass near Earth, instead of just the expected ones. The article is published on the site arXiv preprint server.
Decades after Einstein discovered that his general theory of relativity predicted gravitational waves—ripples propagating through the fabric of spacetime—physicists calculated the expected signatures for a few simple scenarios. One was the passing waveform for black hole mergers, which was the first such wave detected from interferometric data received on September 14, 2015. (The paper wasn’t published until February of the following year.)
By assuming the event that produced the waves, gravitational scientists were able to predict the exact signal that would appear at long-arm laser interferometer facilities such as LIGO (which has two sites in the United States), VIRGO in Italy, and several others around the world.
Observers had to know what to expect so they could train their interferometers to look for what they were looking for, because a passing wave would move the interferometer arms only one-thousandth the width of a proton. Ambient noise, even from passing trucks, could easily cause movement in the arms that had to be filtered out to distinguish a genuine gravitational wave.
Calculations were also performed for neutron star-black hole and neutron star-neutron star mergers. In addition, the signature of continuous gravitational waves produced by rapidly rotating symmetric neutron stars and stochastic gravitational waves originating, for example, from the Big Bang could be deduced from the data. Using these models, more than seven dozen gravitational wave events were detected in total.
But this method does not detect gravitational waves that do not appear as one of the known predictions, called “transients” or “gravitational wave bursts,” which come from unexpected events based on different physics. In addition, current detection methods are too slow.
After a gravitational wave passes, astronomers want to be able to quickly locate its source in order to inform other observatories to look for possible associated electromagnetic or particle events coming from the same source: this is called multi-messenger astronomy.
Electromagnetic radiation, including visible light, and neutrinos are expected during some large-scale and violent astrophysical activities, including routine binary pair mergers. When a potential gravitational wave train is received, processing and communicating with other instruments can currently require hundreds of dedicated processing units and take tens of seconds or even minutes, which is too slow for an “emergency” warning.
In recent years, physicists have tried to improve the limitations of the waveform by using convolutional neural networks (CNNs), a type of specialized deep learning algorithm, to bypass detectors trained to recognize only certain events.
However, to date, programmed CNNs still require an accurate model of the target signal to train and thus fail to detect unexpected sources such as those anticipated by supernova core collapses and long-duration gamma-ray bursts. Unknown physics and computational limitations could ruin any chance of detecting multiple messages.
The researchers set a goal of using a single processor and reporting gravitational wave events in about a second. They developed a multi-component architecture in which one CNN detects simultaneous transients in multiple detectors while a second CNN looks for correlation between detectors to eliminate background noise or coincident problems.
“Our research uses machine learning and aims to help steer ‘traditional’ telescopes towards such a source in seconds,” said Vasileos Skliris of the Institute for Gravity Exploration in the School of Physics and Astronomy at Cardiff University in Wales, UK. “This way, we will be able to extract as much information as possible from these unexpected events.”
The group’s deep learning approach was different from previous methods in one crucial way: Instead of training a CNN to identify specific signal patterns in the data, they created CNNs that could detect coherence in terms of strength and timing between two or more data streams.
The CNNs were then trained using simulated signals and random noise bursts with similar characteristics. By using the same waveform models for the signals and noise, the CNNs were unable to rely on the signal model to make decisions; instead, the CNNs learned to assess the agreement of the detectors with each other, allowing their models to detect gravitational wave transients in real time.
As a test, they ran the observed data for the first two LIGO and VIRGO runs and found good agreement.
“In the 1960s, gamma-ray bursts were the new astrophysical surprise that allowed gamma-ray astronomy to take its first steps,” Skliris said. “Gravitational-wave astronomy is at that same early stage, and we could have an exciting future ahead of us.”
More information:
Vasileios Skliris et al., Real-time detection of unmodeled gravitational wave transients using convolutional neural networks, arXiv (2020). DOI: 10.48550/arxiv.2009.14611
Journal information:
arXiv
© 2024 Science X Network
Quote:Creation of a deep learning algorithm to detect unexpected gravitational wave events (2024, July 25) retrieved July 26, 2024 from https://phys.org/news/2024-07-creation-deep-algorithm-unexpected-gravitational.html
This document is subject to copyright. Apart from any fair dealing for the purpose of private study or research, no part may be reproduced without written permission. The content is provided for informational purposes only.