Time is almost up! There is only one week left to request an invitation to the AI Impact Tour on June 5. Don’t miss this incredible opportunity to explore different methods of auditing AI models. Find out how you can attend here.
Dell reported earnings after the market close on Thursday, beating both profit and revenue estimates, but its results suggest that adoption of AI across its business and among Tier 2 cloud service providers is slower than expected.
Dell stock was down 17.78% in the after-hours after posting a 5.18% loss during the regular trading session, but is still up 86.79% since the beginning of the year.
“Data makes the difference, 83% of all data is on-premises and 50% of data is generated at the edge,” Dell COO Jeff Clarke said during the earnings conference call. “Second, AI gets closer to the data because it is more efficient, effective and secure, and on-premises AI inference can be 75% more cost-effective than the cloud.”
Dell’s current AI strategy is based on the core assumption that businesses will need to deploy infrastructure on-premises rather than in the cloud to take advantage of proximity to data. If this sounds familiar, it should. The company used almost the exact same game during the Great Cloud War.
June 5: the AI audit in New York
Join us next week in New York to engage with top executives and dig deeper into strategies for auditing AI models to ensure optimal performance and accuracy across your organization. Ensure your participation in this exclusive, invitation-only event.
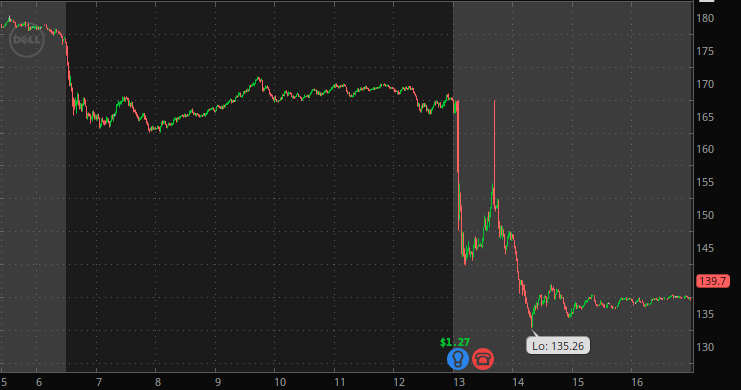
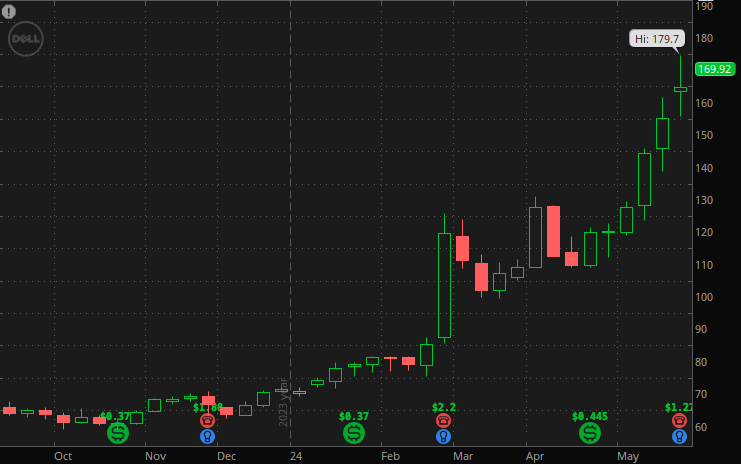
At the time, it was believed that businesses wanted the agility of cloud services, but also control of their own infrastructure.
Ultimately, these purported benefits proved insufficient to resist the inexorable pull of hyperscale clouds for most businesses.
The question that caused Dell to lose $10 billion in market capitalization
Toni Sacconaghi, an analyst at Bernstein, analyzed Dell’s AI server narrative: “So really, the only thing that changed is that you added $1.7 billion in AI servers, and that operating profit remained stable. Does this suggest that AI server operating margins were effectively zero? » Hey, ouch, Toni.
Yvonne McGill, Dell’s CFO, quickly weighed in, saying that “we’ve talked about these AI-optimized servers, which dilute the margin rate, but increase the dollar margin.”
That was CFO language, because you’re absolutely right, Toni, we’re making very little profit on these AI servers right now, but don’t worry.
This is the tried-and-true tactic that Dell has used successfully for decades, selling a loss-making product on the assumption that it will result in a higher margin immediately or in the near future.
Operationally, it’s much easier for customers to deal with a single vendor for purchasing and ongoing support, and the ripple effect is very real.
Specifically, Dell’s margins on networking and storage equipment are significantly higher, and these solutions will likely be bundled with these AI servers, as Jeff Clarke noted: “These (AI) models ) training courses require a lot of data. This data needs to be stored and fed into the GPU with high bandwidth, which is network bound.
Why enterprise AI adoption is still slow
Jeff Clarke’s other remarks give us some clues about the issues holding back AI adoption in business.
Above all, customers are actively trying to determine where and how to apply AI to their business problems. So there are a significant number of services and consultative sales of Dell’s AI solutions.
“Across the company, there are six use cases at the top of almost every discussion,” Clarke said. “These are content creation, support assistance, natural language search, data design and creation, code generation, and document automation. And helping customers understand their data and prepare it for those use cases is what we do today.
This last statement is particularly telling because it suggests how AI projects are still in their early stages.
It also highlights something Clarke doesn’t say directly, which is that AI remains incredibly complicated for the average customer. The data processing, training and deployment pipeline still operates like a fragile Rube Goldberg machine and requires a lot of time and expertise to achieve the promised value. Even just knowing where to start is a problem.
Let’s not forget that businesses faced similar challenges during the Great Cloud War, which posed a barrier to on-premises cloud deployments. An entire cohort of startups has emerged to address complexity issues and replicate the functionality of on-premises public clouds. Most were reduced to ashes when public clouds emerged with their own on-premises solutions, AWS Outposts and Azure Stack.
Yesterday as today, there was the problem of talent. It took an entire decade for cloud skills to diffuse among the technical workforce, and the slow process of migration to the cloud continues today.
Today’s AI stack is even more complex, requiring even deeper domain expertise, another problem that hyperscale clouds are well-positioned to solve with tools and automation deeply integrated into their infrastructures.
Back in the days of the cloud wars, vendors also touted lower costs for on-premises infrastructure, which might even be true in some large-scale cases.
Ultimately, economic considerations prevailed for most companies, and the arguments for cheaper infrastructure were not enough to eliminate operational costs, complexity and close the skills gap.
Even for businesses that are ready to tackle the challenges now, there are supply constraints to overcome. This is because companies are competing for the same Nvidia GPUs on a massive scale and Tier 2 cloud providers are buying on a massive scale.
In this regard, Dell is a truly massive buyer with an excellent track record in balancing the supply of hard-to-source components to many customers. However, Dell customers can currently expect long delivery times for GPU servers.
Dell is playing a long game, but cloud providers could win first
While enterprise AI adoption is still in its early stages, Dell is playing for good.
The company is betting that the need for on-premises AI infrastructure, particularly for latency-sensitive inference workloads, will prove compelling enough for businesses to invest despite the complexity and challenges in of skills.
The strategy is to help companies overcome barriers to AI adoption, even if that means sacrificing short-term margins on GPU servers.
In doing so, Dell leverages its decades of experience solving complex infrastructure problems for customers, as well as its massive scale to keep component supply flowing.
It remains to be seen whether the data problem and edge computing’s attraction to AI will be enough to overcome the inexorable pull of the cloud this time around.
The next few quarters will tell us if Dell’s strategy really works, but this game could already be rigged with cloud providers already offering many enterprise AI offerings running virtually, without the need for much specific equipment from the client side.